Creating competitive advantage with data in retail media
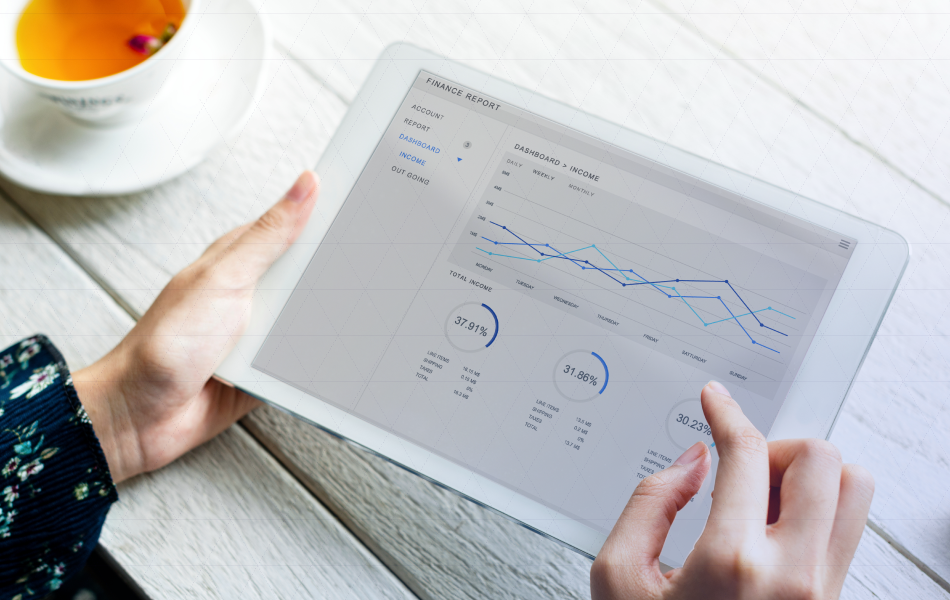
The explosion in digital technologies – and in particular digital commerce – has brands generating an incredible amount of data related to their online and offline retail efforts. While some brands think of this as simply data exhaust, the most sophisticated brands understand that this data is actually fuel for their ever-evolving and growing retail media efforts. In fact, according to HBR and a 2021 study by NewVanatage, only 39% of executives believe their organizations correctly manage data as an asset. And like the best assets, there are compounding effects of investing in that asset early.
These compounding effects are continually being unlocked through advancements in computing technology, machine learning algorithms, and standardizations being implemented across the industry. For brands to best take advantage of these advancements, they must have access to a cleansed, classified, and normalized data set on which to transact. Whether for measuring historic performance and modeling future outcomes or for data sharing via clean rooms, the more accurate and reliable the data asset a brand possesses, the more quickly and reliably they are able to recognize value from that data.
Improved decision-making
Clean, classified, and normalized data ensures that the information used for decision-making is accurate and reliable – especially when making cross-channel investment decisions. One often overlooked aspect of creating a clean dataset is ensuring that data is accurately restated on a regular basis. By eliminating duplicates, errors, and inconsistencies and providing measurements that can be used at the company level (not just the channel level), companies can make more informed decisions based on comparable data.
Enhanced audience targeting
Having access to cleansed data allows brand advertisers to segment and target their audience more effectively. By understanding customer behavior, preferences, and demographics, companies can tailor their advertising campaigns to specific customer segments – such as new-to-brand vs repeat customers – increasing the likelihood of engagement and conversion. This targeted approach leads to higher ROI and outperforms competitors who rely on generic targeting and attribution.
Efficient resource allocation
By understanding which channels, platforms, and ad formats yield the best incremental results, companies can optimize their advertising spend and avoid wasting resources on less performant strategies. This data-driven approach ensures that every dollar spent generates maximum impact, providing a competitive advantage over brands that lack such insights.
Improved measurement and attribution in the customer journey
Normalized and graphed data enables accurate measurement comparison and performance attribution in one channel versus another channel. Companies can track the impact of their campaigns at these touchpoints, identify successful strategies, and make adjustments in real-time. This leads to continuous improvement in the customer’s journey and optimization, helping brands stay ahead of the competition.
Stronger negotiations, partnerships, and collaborations
Unlocking cross-channel measurement with normalized data makes brands and manufacturers attractive partners for other companies and allows them to have data-driven discussions about what a retail trade partner may or may not be providing for them. Brands can collaborate with ad agencies, technology platforms, and publishers more effectively, especially in relation to their Joint Business plans or business reviews. This fosters mutually beneficial partnerships and enables access to exclusive advertising opportunities, further strengthening their competitive position.
Overall, the compounding data advantage contributes to increased effectiveness, customer satisfaction, and ultimately, business success in the dynamic digital retail market. When a brand does this well, it can give them superpowers to optimize and forecast complex cross-channel strategies, and yet, many brands still do this poorly.
Why don’t all brands feel the compounding growth effects of data?
If data is out there, why don’t we see more brands propelled by this asset?
Data fragmentation and silos: Data can be spread across multiple channels and platforms, resulting in data fragmentation. Siloed data makes it challenging to access and integrate information from various sources, hindering the ability to achieve a comprehensive and normalized dataset. This is especially true in advertising in retail media where many channels have closed-loop walled gardens and inconsistent data definitions across channels.
Lack of data infrastructure: Some brands may not have the necessary infrastructure or technology to collect, store, and process data effectively. Data infrastructure and analysis require robust systems, databases, and analytics tools to handle large volumes of data and ensure its quality. Without the proper infrastructure in place, accessing, and managing cleansed and normalized data becomes difficult.
Data privacy, security, and compliance concerns: Brands, retailers, and technology platforms need to adhere to the turning tide in privacy regulations and protect customer data. Ensuring compliance with laws requires implementing stringent data governance practices. These regulations restrict access to certain types of data or require additional measures to anonymize or pseudonymize data, making it more challenging to access the level of data that was previously available.
Limited internal resources and human capital expertise: Preparing data requires dedicated resources and expertise. Many brands may lack the necessary data engineers, data scientists, analysts, or teams specialized in data management and analytics. Without the internal capacity or expertise, brands may struggle to access and process data to a level where it is cleansed, classified, and normalized.
Data availability and partnerships: Acquiring cleansed and normalized data often requires partnerships with data providers, research firms, or third-party vendors. These partnerships may involve costs, licensing agreements, or technical integrations. For example, some Amazon data is only available via direct API access, versus via their brand reporting portal. Brands that lack established relationships or resources to form such partnerships may find it challenging to access high-quality, pre-processed data.
How brands can create a competitive advantage with data?
Creating the dynamic for a compounding data asset in the digital commerce and retail media space requires five key steps:
- Automate data collection across channels
- Automate the cleansing process so that metrics are reported in a consistent format
- Ensure proper classification of your data so that metric definitions align across channels
- Graph data together to enable otherwise siloed data to be analyzed along the same dimensions – for example, mapping campaign spending to a unified SKU allows you to link all the campaign-level marketing performance with all the granular sales performance
- Activating the data with business use cases where learning can be applied to achieve results in short and long term
By setting up a scalable and repeatable process for generating decision-grade data, brands can feel more confident that they can measure the impact of their actions and plan their next steps. If you or your team is looking to unlock growth in your data, learn more about data advantages in retail media and talk to us.