The Endcap and the Polar Bear: A history of attribution in retail marketing
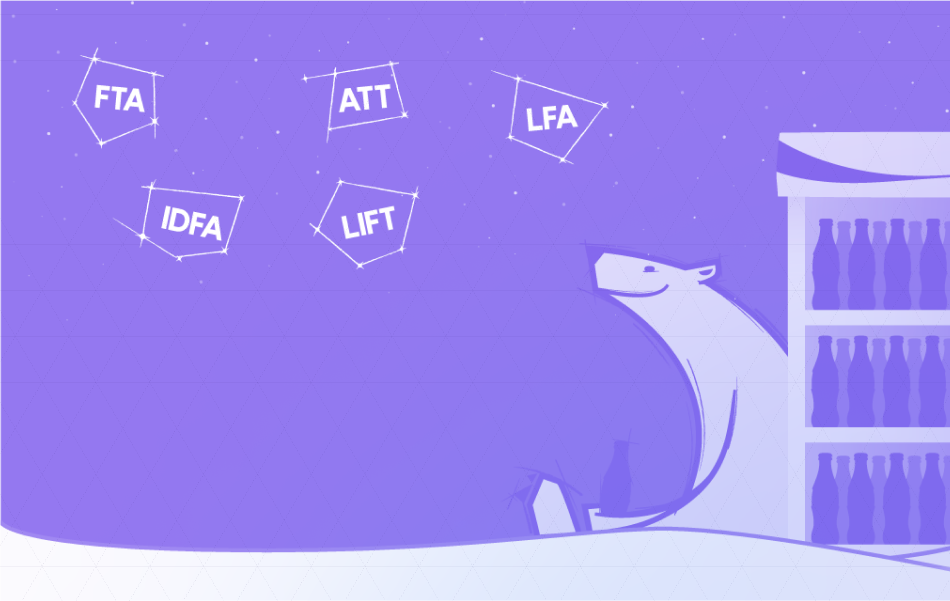
What do an endcap and a polar bear have in common? Both are employed by Coca-Cola to market their products.
The endcap describes the product display at the end of an aisle in a grocery store. It’s designed to grab your attention, and inspire an impulse purchase of a product.
The polar bear, for its part, is ubiquitous in advertising that is often both visually stunning and emotional. Sometimes, the polar bear has a Coca-Cola in its hand and sometimes it doesn’t. Either way, the power of the ad associates the bear with the brand.
Back at Coke HQ, marketers must determine how these parts work together, and how much each part of the strategy is worth investing in. Figuring this out can be a puzzle. Would the sale of one happen without the other? And when that bottle does make it from shelf to cart, which effort deserves the credit?
These are questions that have long bedeviled marketers, whether they are working at startup DTCs or CPG giants. They speak to a common problem: To operate at scale, you also have to understand what worked. When you understand how the different interactions with a brand contributed to a sale, you can invest more in the approaches that are effective, and craft a strategy that accounts for the journey that consumers follow. In turn, you can justify the costs of increasingly expensive and proliferating marketing strategies because you have data to show that they worked.
So how do you do this?
The key to it all is attribution, which aims to determine the cause behind an action. In marketing, attribution seeks to understand how and whether a purchase is the result of advertising and other placements that appear across channels and media. The idea is to determine whether a particular piece of advertising is working, and measure its impact.
The problem is that marketing is a game of influence, while purchases encompass hard data. There is often no one-to-one relationship between the two. For one, a person may be prompted to make a purchase by their own need or preference that has nothing to do with an ad. As the Coca-Cola example illustrates, there are also different types of advertising. Attribution must account for all of these variables, and a number of different approaches have been used since the advent of the supermarket through the internet era.
Let’s review a quick history:
Attribution dates to the 1950s. As national brands like Procter and Gamble emerged out of suburbanization and the growth of the mass market in the 1950s, they sought new ways to determine the effectiveness of the different activities that impacted sales. Michigan State University Professor E. Jerome McCarthy wrote of the 4Ps that comprised the marketing mix: product, price, place, and promotion.
This laid the foundation for marketing mix modeling (MMM), which introduced a probabilistic approach. Popularized in the 1980s and 90s to measure the interplay between TV ads and sales trends, MMM involved experiments that began to test the actions taken by people who came into contact with marketing and those who didn’t. It also took into account a variety of factors, such as the channels where creative was running, things like discounts, and even environmental factors like weather as well as the economy. Applied retroactively, brands use it to understand which channels they should invest in, based on what was shown to be working.
Deterministic models of attribution were thought possible with the arrival of the internet. Interaction with an ad was now measurable. There was data on how many shoppers clicked on an ad, and, with the advent of cookies, it was even possible to determine how many clicks led to a purchase. In turn, purchase data, when combined with characteristics, locations, and other factors, could be harnessed to determine the next set of people that were most likely to purchase a product and market to them, even though they may have never heard of the brand. Harnessed to maximal efficiency by Facebook, this cycle of audience building and retargeting helped to grow a generation of direct-to-consumer brands as an engine of discovery.
With these new tools, it appeared that MMM’s more time-intensive requirements and retrospective vantage point could no longer move at the speed of decision-making. Moreover, the emergence of hard data on behavior led to the belief that the correlation of factors in MMM could be replaced by the ability to show causation between the act of running an ad and the conversion of a sale.
A new generation of attribution models emerged as a result…
First- and last-touch attribution assigned a direct link between interaction with an ad and a purchase. These forms of attribution gave credit for a conversion to either the first touchpoint a person had with a brand or the last place the user visited before arriving at “Buy.” This made a 100% connection between advertising and sales, and for a time, it seemed to solve the problem of attribution.
But this did not account for all of the activities that led to a sale. It was built on the assumption that the only thing influencing a person to make a purchase was an ad that they happened to encounter on the internet—and, in some cases, it was the last ad. In the Coca-Cola example, this would be the equivalent of only giving credit to the endcap.
There are problems with this for a couple of reasons.
It doesn’t account for the way people make choices. People have different reasons for reacting to the endcap. They may have just been thirsty, or they may have entered the store intending to buy a Coke, and happened to come upon the endcap rather than the aisle. There was limited ability to measure whether a sale would have been made with or without the endcap in place.
It is leaving out the role of the polar bear. In other words, it doesn’t take into account all of the other ways Coca-Cola is seeking to ensure you buy that Coke. Ads on TV stations, the internet, and sponsorships of major events all play a role in each stage of decision-making and work together in concert. Consumers move through a funnel as they make purchase decisions, and different forms of marketing are effective at each level. Too often, last-touch attribution treated the endcap as the only influence on a purchase, even if people went there with the intent to buy anyway. It was taking credit for the sun coming up.
This led to a progression of models that aimed to address these deficiencies:
Multi-touch attribution (MTA) was introduced to develop a better understanding of not only the size of the role of these different stages but also how the different channels influenced each other. Reintroducing probabilistic elements, MTA took into account each of the different touchpoints that a customer had on their journey to purchase, and assigned a value to each. This accounted for the different parts of a buying process, and the different places where a brand might advertise.
Lift studies emerged to measure the impact of advertising in a single channel. These studies considered an important question: Would an action have happened even if a person didn’t interact with that ad? They provided measurement of the incremental effect of an ad campaign—meaning, the percentage of the group that was shown an ad, and converted because of it. This could reduce the likelihood of taking credit for the sun coming up, but they were only measuring one channel.
Person-level data and unified measurement then came about to link digital advertising and offline sales. The impact of brand marketing and analysis of the full path-to-purchase entered the equation. Advances in identity systems and cloud computing helped marketers move closer to creating a single view of what was effective.
But these approaches were all powered by key components: third-party cookies from Google and unique identifiers from Apple that allow digital marketing tools to track users across different parts of the web and understand their behavior.

Now, a new era of privacy is blunting those tools.
In April of 2021, Apple introduced key changes in the iOS 14.5 update that made sharing the Identifier for Advertisers, or IDFA, an opt-in rather than a default. In other words, it gave users the upfront choice of opting out, rather than making the tools that allowed tracking a built-in feature. Apple cited a bias toward giving users privacy as it made the change, known as App Tracking Transparency (ATT). Meanwhile, the demise of the third-party cookie is expected to be arriving in the near future. Its official sunset date for cookies in Chrome browsers has been delayed multiple times, and the latest update said it is coming in 2024. Yet Google has advised preparing for the transition, and its moves are inspiring many to build with a privacy-first approach in the meantime.
These changes are striking at the heart of what makes the attribution models developed over the last decade work. ATT’s arrival dealt a particular blow to direct-to-consumer brands that realized profitability through marketing on platforms like Facebook and directing users to their websites to complete conversions. IDFA not only became optional, but access was now behind a prompt in which users were asked if they consented to be tracked. While some users may be fine with this, the more likely answer to whether users wanted to invite an act that sounded invasive was no.
The result for platforms like Facebook was less data from IDFA, and the loss of visibility into users’ activities beyond Facebook. That made it much more difficult to track users and determine whether they bought a product after purchasing an ad. It meant that Facebook had less access to the conversion data that unique identifiers provided to create lookalike audiences for targeting.
Tracking was not only the tool for reaching people with ads. It was also the way that Facebook gained the data to make those ads uniquely effective. The efficiency of this machine gave rise to a generation of new power for brands to connect with the people the exact audiences they wanted to reach at scale, but now they lacked the crucial tools that helped them get there.
What have we learned?
This delivered an important business lesson for many: Don’t get too dependent on any one method. Circumstances can allow you to fly high for a time. But in a highly competitive and transformational field like tech, anything that seems like a silver bullet isn’t likely to last.
In the case of consumer marketing, that was true when considering how many relied on particular platforms like Facebook. Entire DTC playbooks were built around performance marketing on Facebook and converting on Shopify-powered ecommerce stores.
But it was also true of approaches like the last-touch attribution modeling that became so popular over the last decade as a result of the tools that it harnessed. The promise of being able to see exactly which ad was connected to a purchase provided an answer that had long seemed out of reach, namely, which creative and channel delivered conversion. But in truth, it was a reply to the wrong question. The goal should not be to determine whether an ad is working, and optimize for that. Rather, brands must optimize for their growth. The goal is not to make a great Facebook campaign. The goal is to drive sales and to do that, it’s important to look at that campaign in the context of all of a brand’s activities.
This deterministic approach obscured a bigger picture. This became especially important as brands began selling on more channels, creating content on others, and advertising on still more. As we watch consumer behavior shift toward marketplaces and more retailers build their own retail media networks, the need for a brand to continue to expand its presence is only continuing to grow. There’s a recognition that metrics such as return on advertising spend (ROAS) and last-touch attribution are insufficient to measure the totality of a brand’s activity.
In other words, we’re returning to a need for a multilayered model that takes into account not only how a campaign performed, but how an action in one part of the chain impacted all of the others, and where there are opportunities to realize growth that weren’t visible at the point of sale.